Pathway activity inference
scRNA-seq yield many molecular readouts that are hard to interpret by themselves. One way of summarizing this information is by infering pathway activities from prior knowledge.
In this notebook we showcase how to use decoupler
for pathway activity inference with the 3k PBMCs 10X data-set. The data consists of 3k PBMCs from a Healthy Donor and is freely available from 10x Genomics here from this webpage
Note
This tutorial assumes that you already know the basics of decoupler
. Else, check out the Usage tutorial first.
Loading packages
First, we need to load the relevant packages, scanpy
to handle scRNA-seq data and decoupler
to use statistical methods.
[1]:
import scanpy as sc
import decoupler as dc
# Only needed for visualization:
import matplotlib.pyplot as plt
import seaborn as sns
Loading the data
We can download the data easily using scanpy
:
[2]:
adata = sc.datasets.pbmc3k_processed()
adata
[2]:
AnnData object with n_obs × n_vars = 2638 × 1838
obs: 'n_genes', 'percent_mito', 'n_counts', 'louvain'
var: 'n_cells'
uns: 'draw_graph', 'louvain', 'louvain_colors', 'neighbors', 'pca', 'rank_genes_groups'
obsm: 'X_pca', 'X_tsne', 'X_umap', 'X_draw_graph_fr'
varm: 'PCs'
obsp: 'distances', 'connectivities'
We can visualize the different cell types in it:
[3]:
sc.pl.umap(adata, color='louvain')
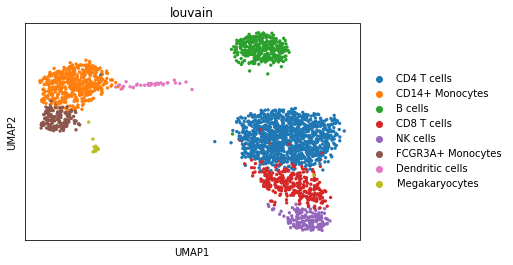
PROGENy model
PROGENy is a comprehensive resource containing a curated collection of pathways and their target genes, with weights for each interaction. For this example we will use the human weights (mouse is also available) and we will use the top 100 responsive genes ranked by p-value. To access it we can use decoupler
.
[4]:
model = dc.get_progeny(organism='human', top=100)
model
[4]:
source | target | weight | p_value | |
---|---|---|---|---|
0 | Androgen | TMPRSS2 | 11.490631 | 0.000000e+00 |
1 | Androgen | NKX3-1 | 10.622551 | 2.242078e-44 |
2 | Androgen | MBOAT2 | 10.472733 | 4.624285e-44 |
3 | Androgen | KLK2 | 10.176186 | 1.944414e-40 |
4 | Androgen | SARG | 11.386852 | 2.790209e-40 |
... | ... | ... | ... | ... |
1395 | p53 | CCDC150 | -3.174527 | 7.396252e-13 |
1396 | p53 | LCE1A | 6.154823 | 8.475458e-13 |
1397 | p53 | TREM2 | 4.101937 | 9.739648e-13 |
1398 | p53 | GDF9 | 3.355741 | 1.087433e-12 |
1399 | p53 | NHLH2 | 2.201638 | 1.651582e-12 |
1400 rows × 4 columns
Activity inference with Multivariate Linear Model
To infer activities we will run the Multivariate Linear Model method (mlm
), but we could do it with any of the other available methods in decoupler
. It models the observed gene expression by using a regulatory adjacency matrix (target genes x pathways) as covariates of the linear model. The values of this matrix are the associated interaction weights. The obtained t-values of the fitted model are the activity scores.
To run decoupler
methods, we need an input matrix (mat
), an input prior knowledge network/resource (net
), and the name of the columns of net
that we want to use.
[5]:
dc.run_mlm(mat=adata, net=model, source='source', target='target', weight='weight', verbose=True)
1 features of mat are empty, they will be removed.
Running mlm on mat with 2638 samples and 13713 targets for 14 sources.
100%|██████████████████████████████████████████████████████████████████████████████████████████████████████████████████████████████████████| 1/1 [00:01<00:00, 1.98s/it]
The obtained scores (t-values)(mlm_estimate
) and p-values (mlm_pvals
) are stored in the .obsm
key:
[6]:
adata.obsm['mlm_estimate']
[6]:
Androgen | EGFR | Estrogen | Hypoxia | JAK-STAT | MAPK | NFkB | PI3K | TGFb | TNFa | Trail | VEGF | WNT | p53 | |
---|---|---|---|---|---|---|---|---|---|---|---|---|---|---|
AAACATACAACCAC-1 | -0.250004 | 1.082446 | -0.283717 | 0.018847 | -1.101526 | -1.425420 | -0.079885 | -0.806441 | -1.146634 | 0.685259 | -0.574002 | 0.138756 | 0.127025 | 0.056324 |
AAACATTGAGCTAC-1 | 0.055699 | 1.808353 | -0.639859 | 0.547163 | 0.053690 | -2.664019 | 0.503815 | -0.056263 | -1.252882 | -0.669461 | 0.665907 | 0.348851 | -0.883580 | -1.075813 |
AAACATTGATCAGC-1 | -1.300669 | 1.093902 | -1.423962 | 0.862988 | 0.770645 | -1.604121 | -0.089351 | -0.293437 | -0.817603 | 0.895554 | 0.004694 | 0.213210 | -0.377153 | 0.001996 |
AAACCGTGCTTCCG-1 | -1.108973 | 0.623916 | -0.533218 | 0.456434 | 5.369927 | -1.157837 | -1.695246 | -0.328195 | -0.652306 | 1.713096 | -0.190242 | -0.439180 | -0.290556 | -1.360429 |
AAACCGTGTATGCG-1 | -0.020885 | -0.934698 | 0.113905 | 0.480329 | 2.008395 | 1.355708 | -0.277678 | 0.018336 | -0.362728 | -0.004614 | 0.700825 | -0.166572 | -1.324292 | -0.742359 |
... | ... | ... | ... | ... | ... | ... | ... | ... | ... | ... | ... | ... | ... | ... |
TTTCGAACTCTCAT-1 | -1.310079 | 1.646397 | -0.519146 | 0.867002 | 5.929371 | -1.278792 | -2.046493 | 0.863211 | -0.123756 | 2.284416 | -0.393778 | 0.147630 | 0.384334 | -0.721629 |
TTTCTACTGAGGCA-1 | -0.084679 | -0.269608 | -0.333985 | 0.554965 | 1.318137 | -0.552446 | -0.633511 | 0.272859 | -1.498412 | 0.175075 | 0.222500 | 0.315759 | -0.354096 | -0.359570 |
TTTCTACTTCCTCG-1 | -0.381286 | 0.296119 | -0.583498 | -0.474597 | 0.406432 | -0.774971 | -1.212176 | -1.746175 | -0.688630 | 1.496862 | 1.035139 | 0.253294 | -0.590249 | -0.908120 |
TTTGCATGAGAGGC-1 | -0.077524 | 1.164005 | -0.051290 | -0.926941 | -1.005181 | -1.171102 | -0.631157 | 0.763098 | -0.933493 | 0.305002 | 2.437439 | -0.133605 | 0.625714 | -0.178558 |
TTTGCATGCCTCAC-1 | -0.211075 | 0.498874 | 0.701253 | 0.233277 | 1.078380 | -0.977642 | -0.786710 | -1.324148 | -0.756899 | 1.250499 | 0.312962 | 0.160146 | 0.040263 | -1.152789 |
2638 rows × 14 columns
Note: Each run of run_mlm
overwrites what is inside of mlm_estimate
and mlm_pvals
. if you want to run mlm
with other resources and still keep the activities inside the same AnnData
object, you can store the results in any other key in .obsm
with different names, for example:
[7]:
adata.obsm['progeny_mlm_estimate'] = adata.obsm['mlm_estimate'].copy()
adata.obsm['progeny_mlm_pvals'] = adata.obsm['mlm_pvals'].copy()
adata
[7]:
AnnData object with n_obs × n_vars = 2638 × 1838
obs: 'n_genes', 'percent_mito', 'n_counts', 'louvain'
var: 'n_cells'
uns: 'draw_graph', 'louvain', 'louvain_colors', 'neighbors', 'pca', 'rank_genes_groups'
obsm: 'X_pca', 'X_tsne', 'X_umap', 'X_draw_graph_fr', 'mlm_estimate', 'mlm_pvals', 'progeny_mlm_estimate', 'progeny_mlm_pvals'
varm: 'PCs'
obsp: 'distances', 'connectivities'
Visualization
To visualize the obtained scores, we can re-use many of scanpy
’s plotting functions. First though, we need to extract the activities from the adata
object.
[8]:
acts = dc.get_acts(adata, obsm_key='mlm_estimate')
acts
[8]:
AnnData object with n_obs × n_vars = 2638 × 14
obs: 'n_genes', 'percent_mito', 'n_counts', 'louvain'
uns: 'draw_graph', 'louvain', 'louvain_colors', 'neighbors', 'pca', 'rank_genes_groups'
obsm: 'X_pca', 'X_tsne', 'X_umap', 'X_draw_graph_fr', 'mlm_estimate', 'mlm_pvals', 'progeny_mlm_estimate', 'progeny_mlm_pvals'
dc.get_acts
returns a new AnnData
object which holds the obtained activities in its .X
attribute, allowing us to re-use many scanpy
functions, for example let’s visualise the Trail pathway:
[9]:
sc.pl.umap(acts, color='Trail', vcenter=0, cmap='coolwarm')
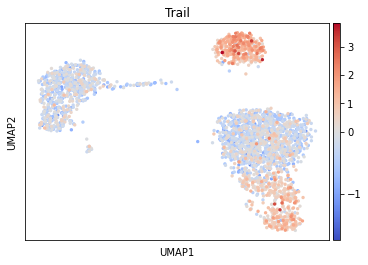
It seem that in B and NK cells, the pathway Trail, associated with apoptosis, is more active.
Exploration
With decoupler
we can also see what is the mean activity per group:
[10]:
mean_acts = dc.summarize_acts(acts, groupby='louvain', min_std=0)
mean_acts
[10]:
Androgen | EGFR | Estrogen | Hypoxia | JAK-STAT | MAPK | NFkB | PI3K | TGFb | TNFa | Trail | VEGF | WNT | p53 | |
---|---|---|---|---|---|---|---|---|---|---|---|---|---|---|
B cells | -0.465011 | 0.342595 | -0.415550 | -0.124931 | 0.823214 | -0.876431 | -0.587007 | -0.215912 | -0.879469 | 0.644808 | 1.158729 | -0.182334 | -0.217285 | -0.567988 |
CD14+ Monocytes | -0.753841 | 1.052265 | -0.489119 | 0.773133 | 2.393195 | -1.379257 | -0.983988 | -0.230894 | -0.771927 | 1.477719 | -0.267905 | -0.276274 | 0.010432 | -0.572246 |
CD4 T cells | -0.735975 | 0.412647 | -0.440776 | 0.292130 | 0.953193 | -0.843468 | -0.494001 | -0.651749 | -0.888945 | 0.916335 | -0.272181 | 0.081154 | -0.226721 | -0.627953 |
CD8 T cells | -0.726172 | 0.455278 | -0.407548 | 0.322529 | 1.111638 | -0.879143 | -0.753404 | -0.353885 | -0.990159 | 1.231635 | 0.296404 | 0.095871 | -0.129972 | -0.673387 |
Dendritic cells | -0.684471 | 1.089961 | -0.636636 | 0.789944 | 3.087430 | -1.581596 | -0.516469 | -0.536889 | -0.765263 | 0.860534 | -0.337834 | -0.456041 | 0.187420 | -0.607646 |
FCGR3A+ Monocytes | -0.864837 | 1.320364 | -0.632086 | 0.927257 | 3.654879 | -1.635532 | -1.153630 | -0.113269 | -1.156720 | 1.760448 | -0.229468 | -0.333119 | -0.358097 | -0.633038 |
Megakaryocytes | -0.536795 | 2.360259 | -0.378290 | 1.376318 | 0.080829 | -2.250954 | -0.627291 | -1.006189 | 0.010520 | 0.661547 | -0.045912 | 0.003110 | 0.962199 | -0.311917 |
NK cells | -0.691467 | 0.563583 | -0.209429 | 0.389797 | 2.215324 | -1.014608 | -1.160875 | -0.101439 | -1.083613 | 1.240734 | 0.733171 | 0.264418 | -0.094835 | -0.708889 |
We can visualize which group is more active using seaborn
:
[11]:
sns.clustermap(mean_acts, xticklabels=mean_acts.columns, vmin=-2, vmax=2, cmap='coolwarm')
plt.show()
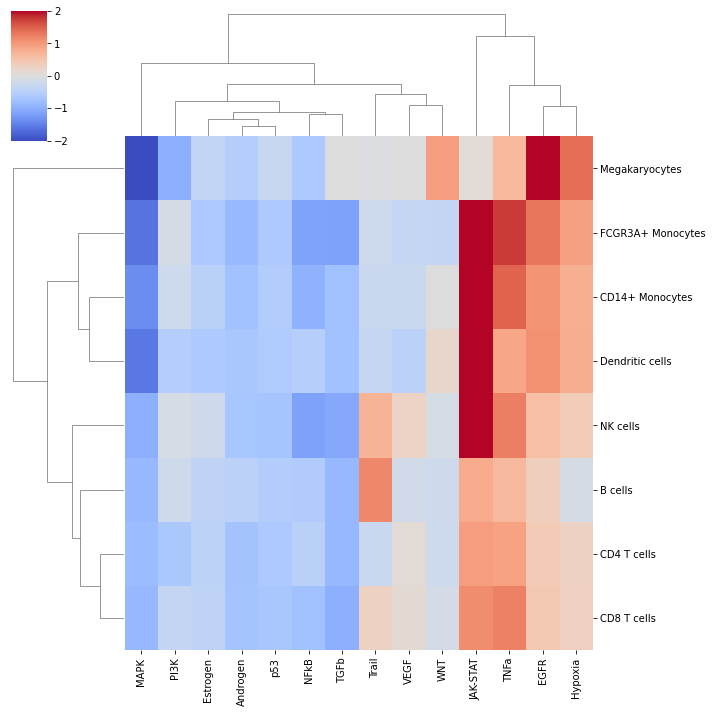
In this specific example, we can observe that WNT to be more active in Megakaryocytes, and that Trail is more active in B and NK cells.
Note
If your data consist of different conditions with enough samples, we recommend to work with pseudo-bulk profiles instead. Check this vignette for more informatin.